Ian Pan
PGY-5 Radiology Resident at Brigham and Women's Hospital.
Kaggle Competitions Grandmaster.
BS, MA, MD from Brown University.
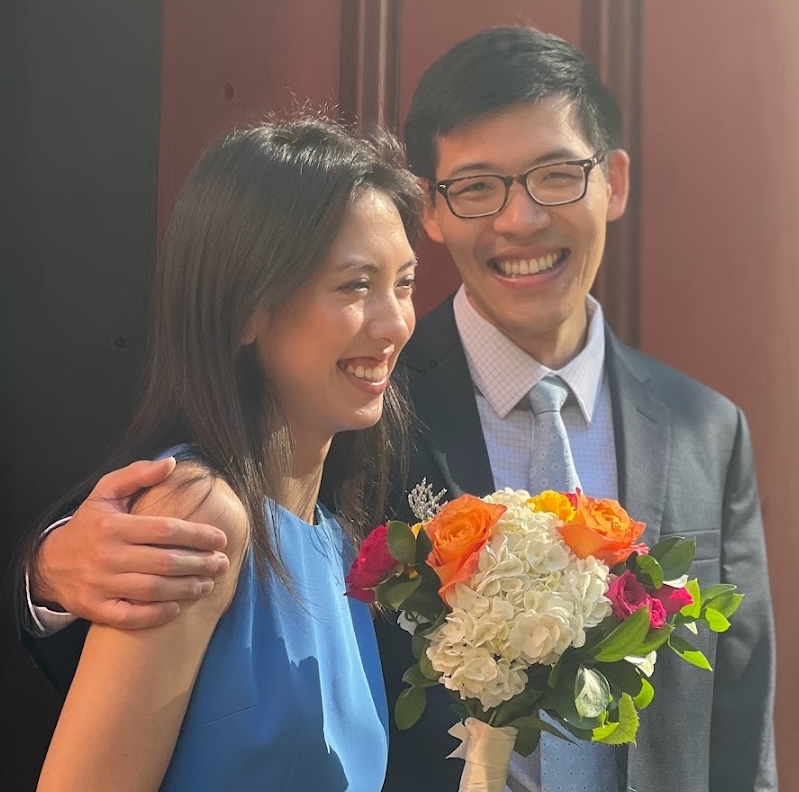
Hi, I’m Ian. I’m currently a radiology resident in the Brigham and Women’s Hospital/Harvard Medical School Diagnostic Radiology Residency Program, where I also served as Chief Resident from 2023-2024. I will be staying at Brigham as a neuroradiology fellow for 2025-2026.
I’m in interested in applications of artificial intelligence to healthcare, specifically radiology and medical imaging. I have published several manuscripts and continue to work on various projects in this area. I’m also an avid Kaggler and the first physician to earn the title of Kaggle Competitions Grandmaster, focusing mainly on competitions related to medical imaging. At my peak, I was top 10 in the world (#9).
In my free time, I like to eat, run, and watch K-dramas. I also enjoy spending time with my amazing retina surgeon wife.
news
Nov 20, 2024 | My team won 2nd place in the RSNA Lumbar Spine Degenerative Classification AI Challenge hosted on Kaggle |
---|---|
Nov 04, 2024 | I won 1st place in the MIDRC XAI Grand Challenge |
May 08, 2024 | I received an RSNA Research Resident Grant for my project Artificial Intelligence for Longitudinal Segmentation and Tumor Risk Prediction of IDH-Mutant Gliomas |